AI models are rapidly evolving, with new models and improved versions being released at a breakneck pace. While the top language models offer comparable overall performance, some models seem to hold an edge in specific types of tasks.
In the process of developing SmartResolve — Instabug’s AI-powered code fix generation feature — we performed an in-depth evaluation of several leading large language models (LLMs) to determine the best-performing ones. Our evaluation focused on how well the AI model is able to analyze crash reports, identify their root cause, and generate a code fix.
In this post, we share the platform-specific performance for the following AI models when generating code fixes for mobile crashes across iOS and Android:
- OpenAI GPT-4o, o1, o3-mini
- Gemini 1.5 Pro
- Anthropic Claude Sonnet 3.5 V1, Claude Sonnet 3.5 V2, Claude Haiku 3.5 V1
- Meta LLama 3.3 70B
Evaluation Methodology & Criteria
To assess the capabilities of each LLM, we conducted standardized tests using a diverse dataset of real-world crashes and their corresponding fixes. These fixes were introduced and reviewed by experienced mobile developers on both iOS and Android.
While SmartResolve involves multiple processing steps, including an advanced retrieval-augmented generation (RAG) pipeline, this report focuses solely on the models’ ability to generate correct, human-like code fixes.
Each generated fix was evaluated against five criteria:
- Correctness: How effectively the generated fix resolves the crash and prevents its regression.
- Similarity: How closely the fix resembles a human-written solution.
- Depth: How well the fix identifies and addresses the root cause of the crash.
- Relevance: How well the fix aligns with the crash stack trace and root cause.
- Coherence: The clarity, structure, and logical flow of the fix.
Each model received a score out of 100 for each criterion, then an overall accuracy score was calculated using weighted contributions from each category. These weights were designed to reflect the importance of each factor in determining the overall effectiveness of generated fixes.
- Correctness: 40%
- Similarity: 30%
- Depth: 10%
- Relevance: 10%
- Coherence: 10%
Model Evaluations
OpenAI GPT-4o
iOS weighted total: 59.81%
Android weighted total: 48.97%

While GPT-4o scored well on both platforms, its performance on iOS was significantly better, delivering greater coherence and correctness. This positions it as a reliable candidate for structured tasks that require consistent performance.
On the other hand, GPT-4o faced more challenges on Android and struggled with diminished relevance and depth, potentially compromising the quality of code fixes.
Additionally, the model displayed high consistency in its results and faster response times across multiple evaluations. While these factors may not directly impact the effectiveness of the generated fixes, they significantly influence the model's scalability and effectiveness in real-world production scenarios like SmartResolve.
OpenAI o1
iOS weighted total: 61.79%
Android weighted total: 26.31%

Again, the o1 model exhibits an overwhelming advantage on the iOS platform, excelling in the correctness, coherence, and relevance of its generated responses. However, on Android, o1 struggled much more than GPT-4o and scored significantly lower across all evaluated metrics, dismissing it as a suitable choice for generating crash fixes on the platform.
Although not one of the evaluation criteria, a major observation during the evaluation was the substantial latency in o1's response times. In 50% of cases, particularly within the Android evaluations, o1 failed to provide any response at all. This issue persisted even when the model was repeatedly evaluated on the same dataset.
In a production environment where scalability and reliability are paramount, o1's unpredictable and slow response times would pose significant challenges and hinder its effectiveness even on iOS.
OpenAI o3-mini
iOS weighted total: 59.07%
Android weighted total: 46.38%

Continuing the trend, the performance of o3-mini on iOS was significantly better than on Android, particularly in generating correct and coherent responses, making it a strong candidate for resolving iOS crashes. While o3-mini’s performance on Android devices was acceptable, the correctness, depth, and relevance of its responses were relatively modest.
This indicates that o3-mini may not be the best choice to address Android crashes, especially ones that are more complex and require intricate code fixes.
Gemini 1.5 Pro
iOS weighted total: 58.53%
Android weighted total: 51.41%

Gemini 1.5 Pro delivers solid overall performance across both platforms, particularly excelling on iOS in generating correct and coherent responses. The model’s results on Android show slightly stronger performance in relevance, but it lags behind in depth and correctness.
Gemini 1.5 Pro’s capacity for a significantly larger context window compared to other models sounded like a great advantage, especially for tasks involving extensive codebases. However, in practical testing, the model's performance deteriorated as the context window expanded due to increased hallucinations, affecting its overall performance across all metrics.
While a larger context window can be beneficial, there are also potential drawbacks, and careful tuning and management of the context window are crucial for optimal performance.
Claude Sonnet 3.5 V1
iOS weighted total: 58.33%
Android weighted total: 55.56%

Claude 3.5 Sonnet V1 demonstrates strong performance on both platforms, with iOS exhibiting a slight advantage in coherence and correctness. While Android results offer marginally better relevance, it slightly underperforms in coherence, making it slightly less consistent..
Overall, Sonnet strikes a good balance across both platforms, making it a versatile option. It is particularly well-suited for applications that require a blend of structured reasoning and relevance.
Claude Sonnet 3.5 V2
iOS weighted total: 49.87%
Android weighted total: 47.56%
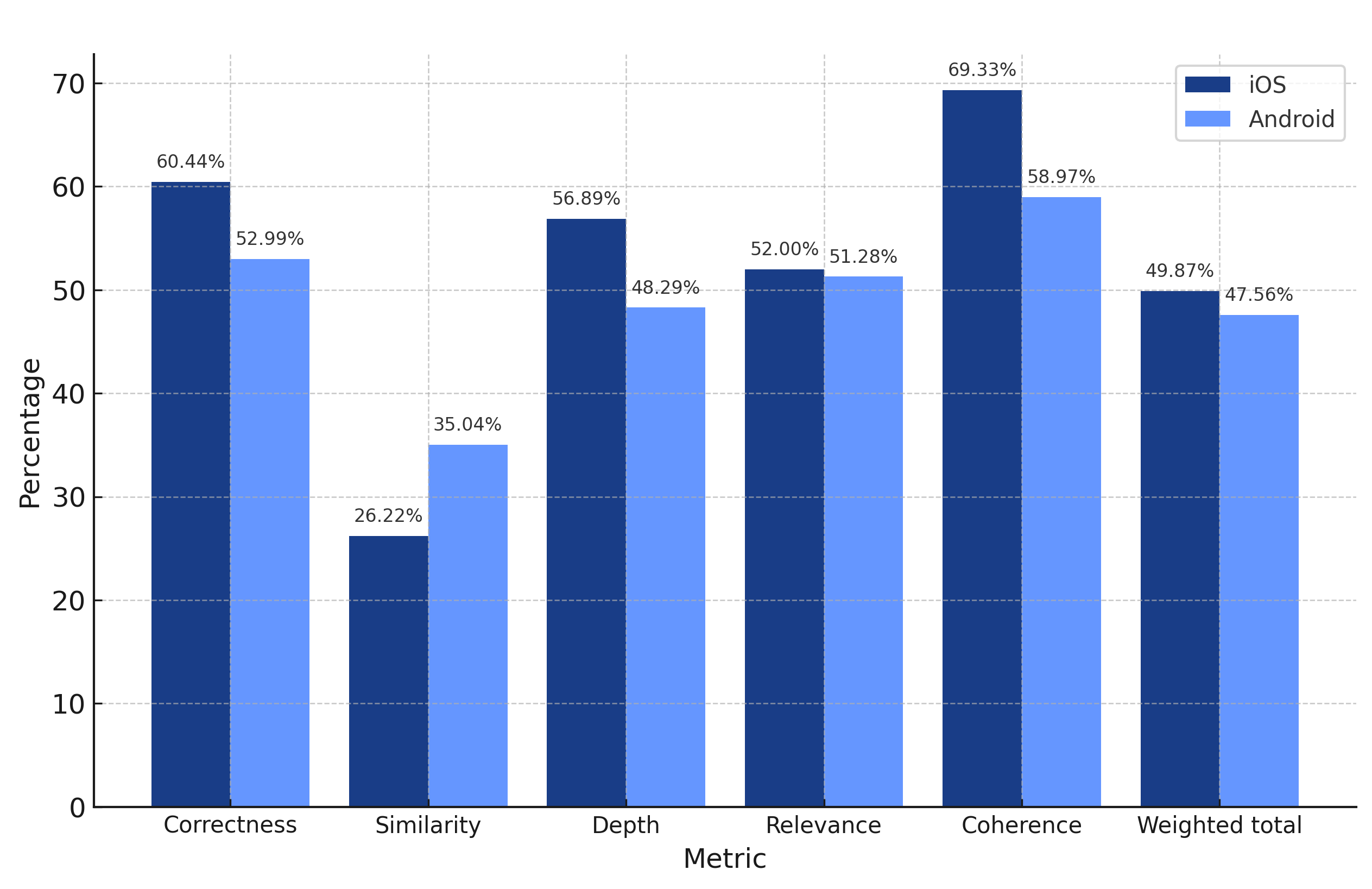
Claude 3.5 Sonnet V2 delivers comparable performance on both platforms but, surprisingly, lacks the depth and correctness of the other models from Anthropic. We were not expecting a groundbreaking improvement over V1, but it was quite shocking to see V2 perform significantly worse.
While V2’s overall performance was not terrible, it was comfortably beaten across the board by both of Anthropic’s other models in this evaluation.
Claude Haiku 3.5
iOS weighted total: 57.47%
Android weighted total: 57.68%

Claude 3.5 Haiku V1 is nearly identical in performance across both platforms, with Android performing marginally better in relevance. This model is well-balanced and can be used effectively on both platforms without noticeable trade-offs.
Claude Haiku was one of the earliest models that we tested in our benchmarking activities, and we thought it would be overtaken by Claude Sonnet 3.5, but its consistency across Andriod and iOS, as well as going toe to toe with Claude Sonnet 3.5, was very surprising.
LLama 3.3 70B
iOS weighted total: 38.75%
Android weighted total: 16.30%

LLaMA 3 70b Instruct V1 disappointed across both platforms, with extremely poor performance on Android. Its low coherence and correctness make it unsuitable for fix generation, and even its iOS performance is subpar compared to other models.
A big part of LLama’s poor performance can be attributed to its struggle to provide a reliable, structured JSON output, which is crucial in building scalable, production-ready software around it.
Overall Results
iOS Results
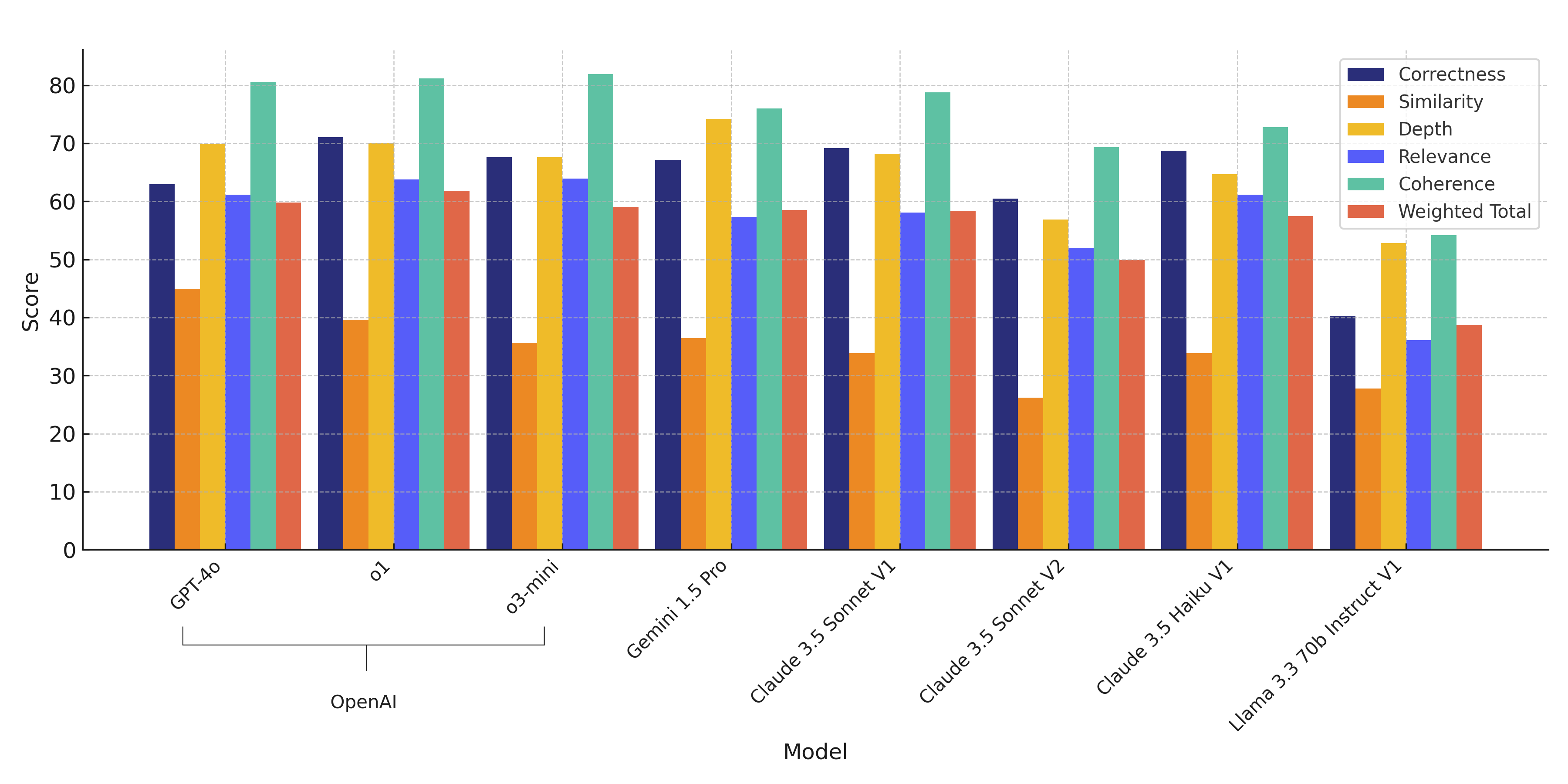
Android Results
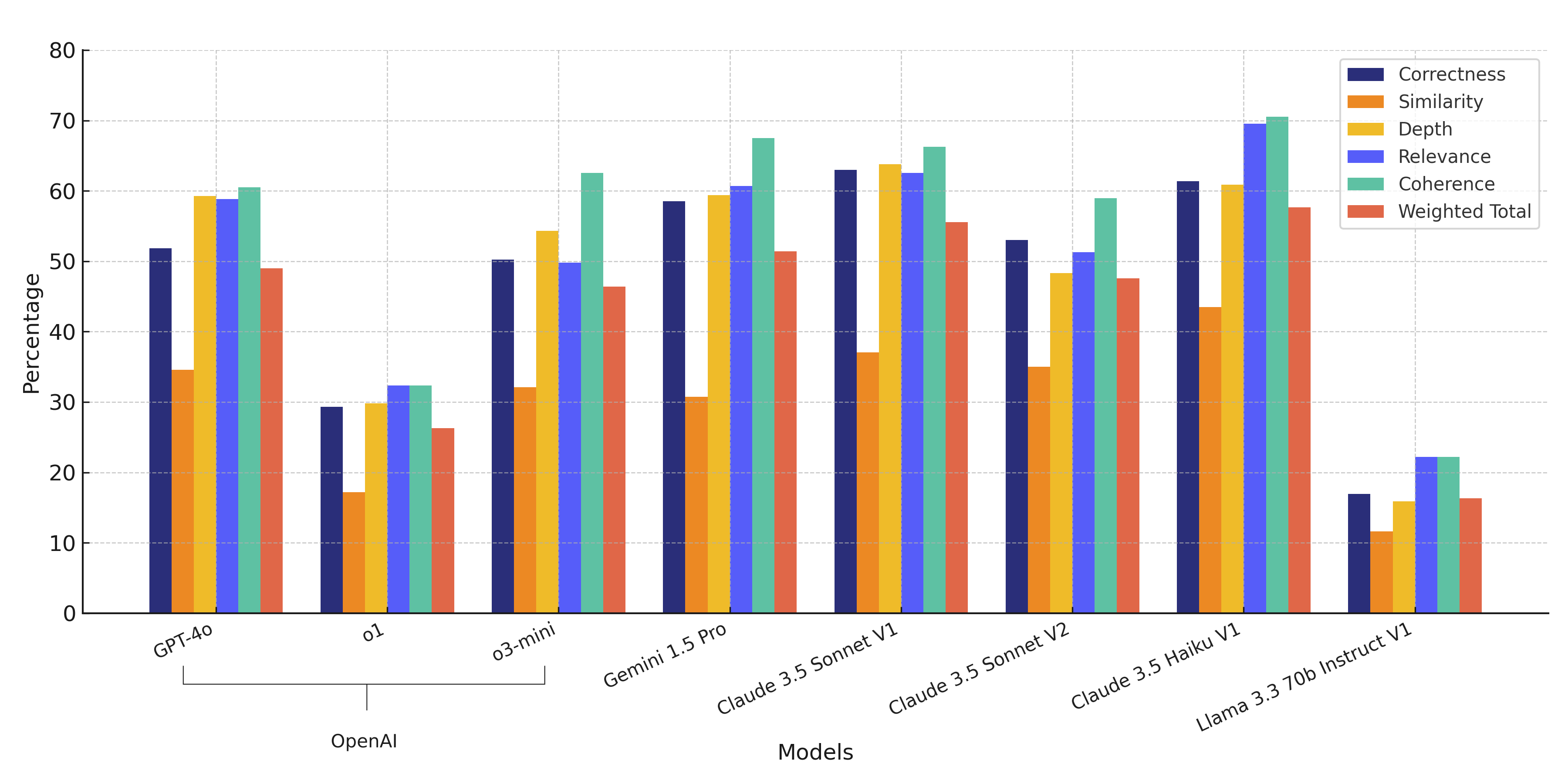
Conclusion
The results highlight that most models performed better on iOS, with GPT-4o, Claude 3.5 Haiku V1, and Claude 3.5 Sonnet V1 emerging as the strongest contenders due to their consistency and structured outputs. Conversely, models like LLaMA-3-70b and OpenAI o1 struggled significantly, particularly on Android, due to poor correctness, frequent failures, and slow response times.
For SmartResolve’s production use, a hybrid model selection strategy emerged as the ideal option—leveraging high-coherence models like GPT-4o for structured responses while integrating stable models like Claude Haiku 3.5 and Claude Sonnet 3.5 for balanced performance across platforms.
With new models like DeepSeek R1 and Claude Sonnet 3.7 entering the market and providing better performance, we’re continuously updating our evaluations to identify the best model for each mobile platform and crash type, ensuring SmartResolve remains at the forefront of AI-powered mobile crash resolution.
Stay tuned for more LLM benchmarking evaluations.
Learn more:
- The 7 Best AI-Powered AppSec Tools You Can’t Ignore
- How to Use AI for Onboarding: 8 Tools to Boost Retention
- Top AI and ML Mobile Testing Tools for Apps
- AI-Enabled Mobile Observability: A Future of Zero-Maintenance Apps
Instabug empowers mobile teams to maintain industry-leading apps with mobile-focused, user-centric stability and performance monitoring.
Visit our sandbox or book a demo to see how Instabug can help your app